Research
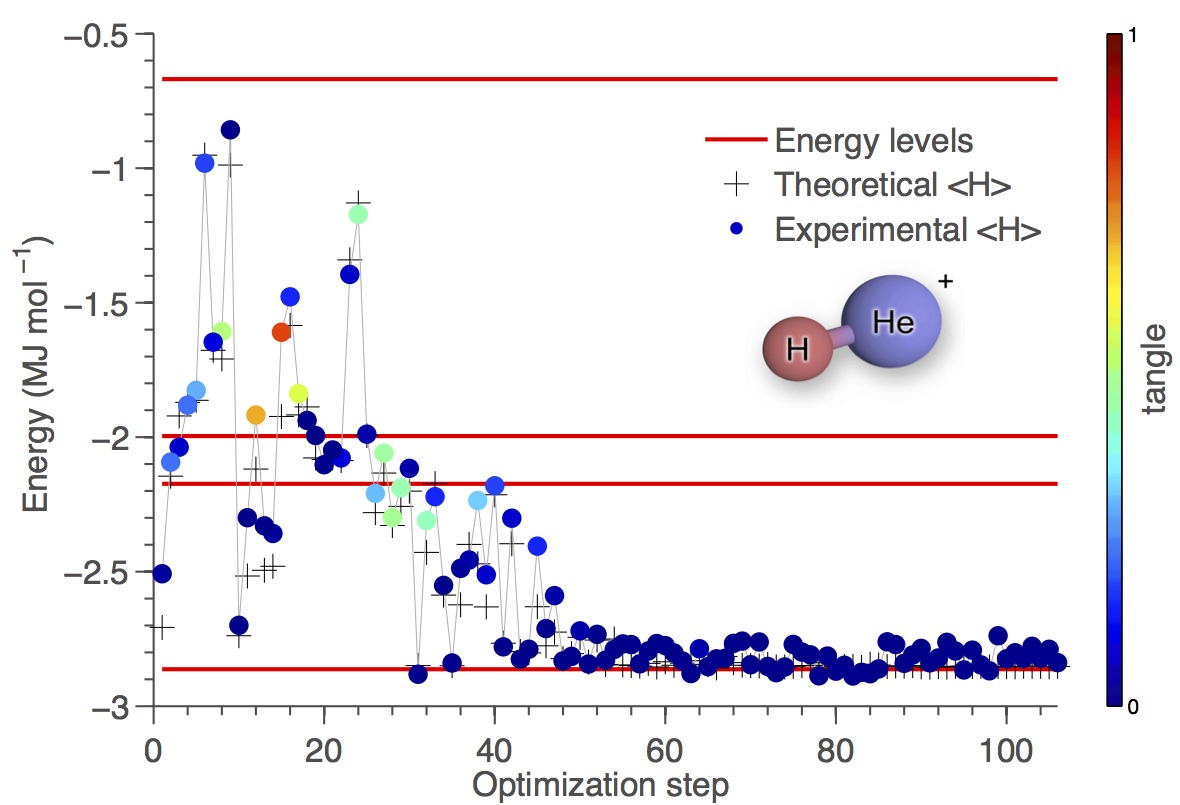
Quantum information processing and quantum simulations with photons
Quantum information science provides new paradigms of communication, measurement and computation. Some of the most startling future quantum technologies include quantum key distribution, which offers perfectly secure communication; quantum metrology, which allows more precise measurements than could ever be achieved without quantum mechanics and quantum computers, which promise exponentially faster operation for particular tasks. Particularly appealing today are quantum simulators, where one controllable quantum system is used to efficiently investigate the behaviour and properties of another, less accessible one. Quantum simulators hold the promise of tackling problems that are too demanding for classical computers but would require far less resources than a full-scale quantum computer.
Integrated photonic quantum circuits
Recent quantum optical work has highlighted the promise of monolithic integrated optics for quantum information science. We have begun to address the integration of superconducting single photon detectors and non-linear single photon sources. We have addressed miniaturisation using multimode interference architectures to directly implement NxN Hadamard operations. We take advantage of the photonic technology to demonstrate high-fidelity realizations of key quantum photonic circuits , the building blocks of future quantum simulators for physical, chemical and biological systems and for fundamental aspects of quantum measurement.
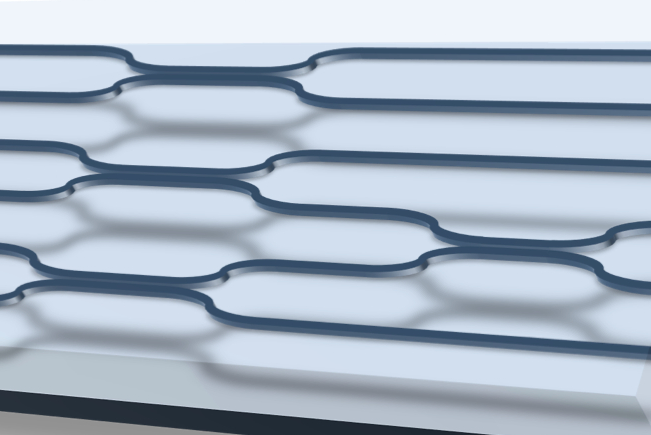
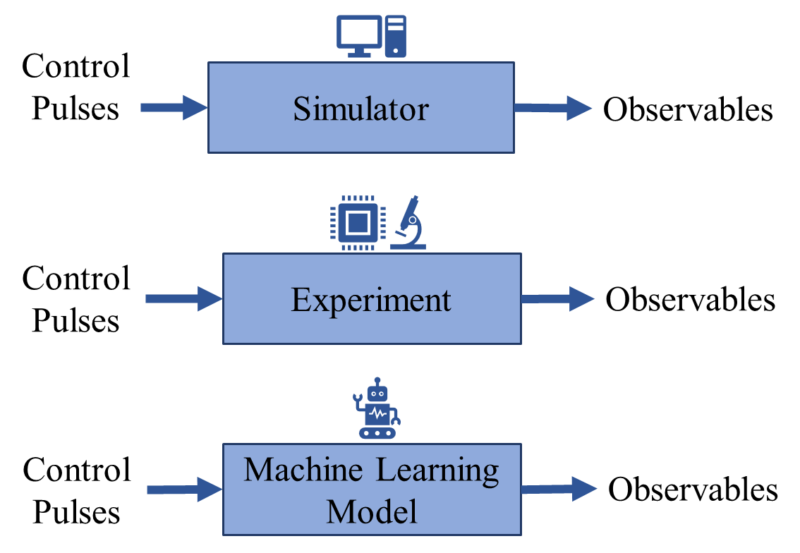
Quantum Control
Quantum noise is an obstacle that prevents quantum systems, which are being developed as future quantum technologies, from fulfilling their game-changing potential. Understanding the characteristics of this noise is crucial to optimising a system’s performance. Currently available control methods strongly rely on assumptions on the noise (e.g. Markovian, quasi-static, weakly-coupled to the system), and the control signals (e.g. infinite bandwidth and/or power). We develop machine-learning based techniques that aim to characterize and control noisy quantum systems under realistic experimental conditions.
Machine learning for quantum information science and technology
Explaining quantum correlations through evolution of causal models, Robin Harper, Robert J. Chapman, Christopher Ferrie, Christopher Granade, Richard Kueng, Daniel Naoumenko, Steven T. Flammia, Alberto Peruzzo, Phys. Rev. A 95, 042120 (2017)
Experimental Demonstration of Self-Guided Quantum Tomography, Robert J. Chapman, Christopher Ferrie, Alberto Peruzzo, Physical Review Letters 117, 040402 (2016)
A variational eigenvalue solver on a photonic quantum processor, Alberto Peruzzo, Jarrod McClean, Peter J. Shadbolt, Man-Hong Yung, Xiao-Qi Zhou, Peter Love, Alan Aspuru-Guzik, Jeremy L. O’Brien, Nature Communications 5:4213, (2014)
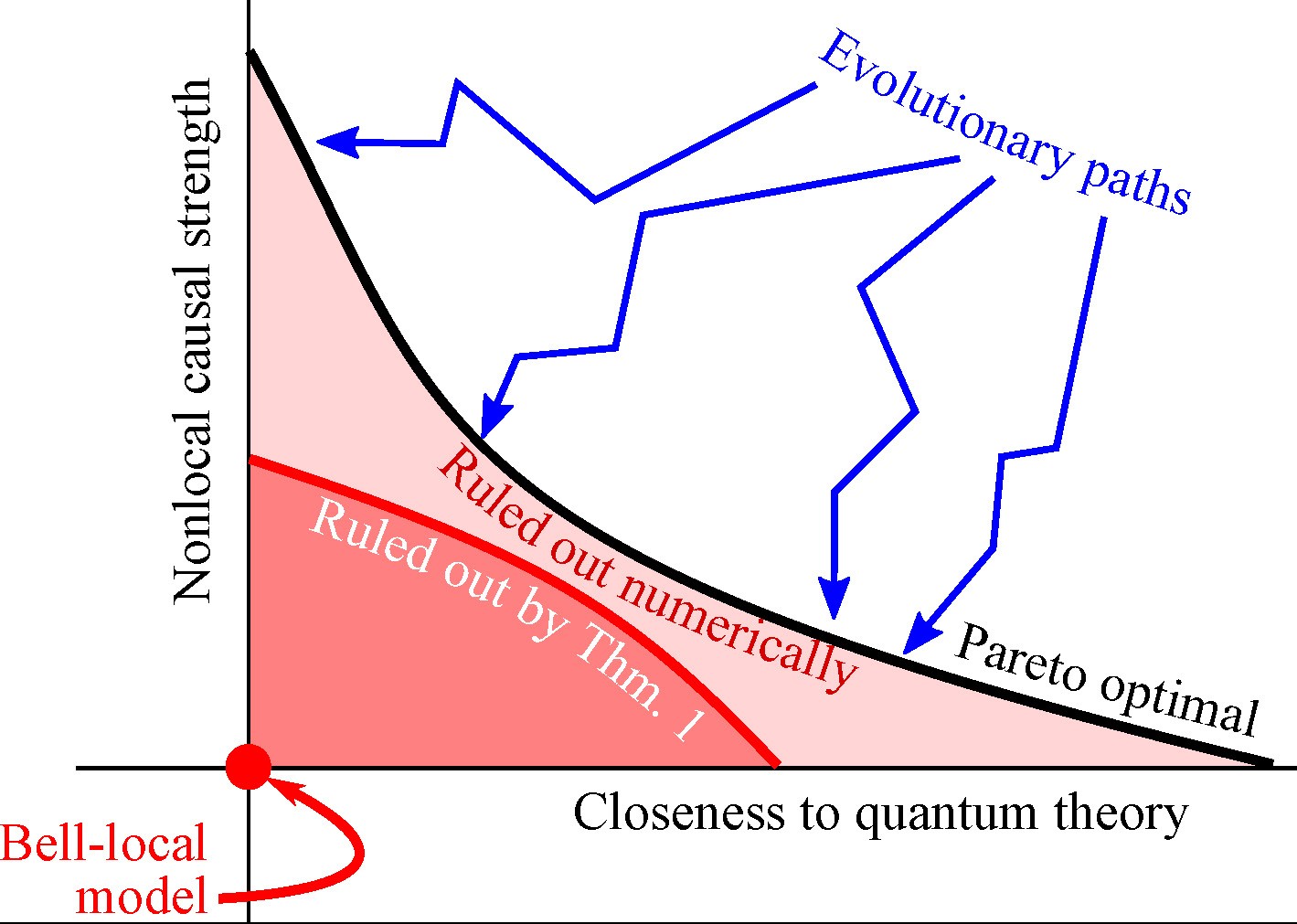